In the fast-paced world of artificial intelligence (AI), Vertical Large Language Models (LLMs) are changing the game. These specialized models have the unique ability to transform how we apply AI in various industries. In this blog post, I aim to explore the captivating world of Vertical LLMs, highlight their applications, and evaluate the benefits and challenges they present. I will also look ahead to emerging trends that may influence the future of Vertical LLMs in AI.
Understanding Vertical LLMS
Vertical LLMs are designed to operate in specific industries or domains, unlike general LLMs which try to cover a wide range of topics. This focus enables Vertical LLMs to provide more accurate and context-specific insights, enhancing both user experience and overall performance.
For example, while models like OpenAI's GPT-3 can generate text on countless subjects, they may struggle with specialized tasks. Vertical LLMs, however, are created with training data that concentrates on the nuances and terminology relevant to a specific field. This makes them more effective in industries such as healthcare, finance, and law.
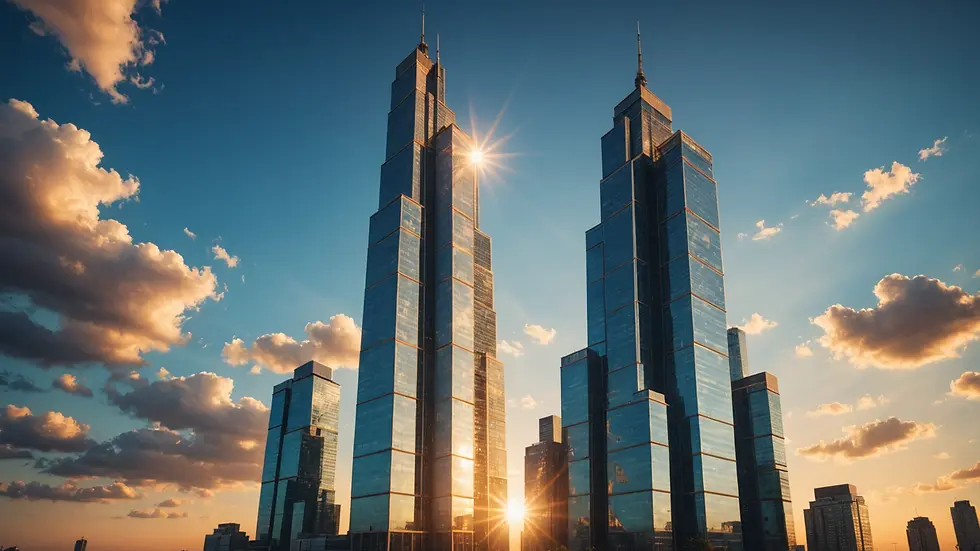
Applications in AI
The potential applications of Vertical LLMs are extensive and impactful. Here are a few significant areas where these models are making strides:
1. Healthcare
In healthcare, Vertical LLMs can dramatically improve patient care and clinical research. By training models with extensive datasets that include medical terminology, treatment protocols, and patient interactions, these LLMs can help generate precise medical documentation. For instance, studies show that AI models can reduce documentation time for healthcare professionals by up to 40%, allowing them to focus more on patient care. Furthermore, these models can assist in diagnostics and offer tailored therapy recommendations based on individual patient data.
2. Finance
Vertical LLMs also have transformative applications in the financial sector. By specializing in areas like fraud detection and risk assessment, these models can analyze transaction patterns and stay compliant with regulations more effectively than general models. A notable example includes banks using AI to spot fraudulent transactions instantly, leading to a 30% decrease in financial losses due to fraud over two years.
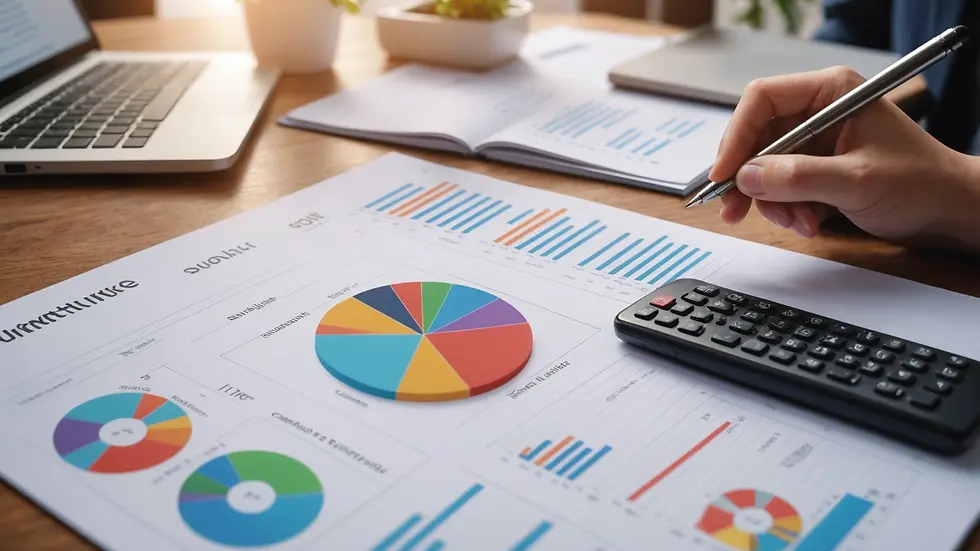
3. Legal
Vertical LLMs are also streamlining workflows in the legal profession. Equipped with training data from case law, legal documents, and statutes, these models can aid lawyers in drafting briefs and conducting legal research. They save time by predicting case outcomes based on historical legal data, enabling professionals to focus on strategy. Reports note that using AI for legal research can cut time spent on this task by approximately 50%, resulting in more efficient case management.
4. Education
In education, Vertical LLMs can foster personalized learning experiences. By providing tailored quizzes, study materials, and feedback based on student performance, these models enhance engagement and understanding. Schools implementing AI-powered learning systems have observed improvement rates in student grades by as much as 25%, showcasing the potential for targeted growth and educational success.
Advantages and Limitations
While Vertical LLMs offer numerous benefits, it is essential to consider their challenges. Below are important aspects to keep in mind:
Advantages
Improved Accuracy: Vertical LLMs utilize domain-specific insights, leading to more relevant and precise outcomes. This specialization enables these models to produce context-aware responses.
Increased Efficiency: By concentrating on specific sectors, Vertical LLMs can generate relevant content in a fraction of the time traditional models require. This efficiency is especially valuable in industries with tight deadlines.
Limitations
Data Quality: A primary challenge in developing Vertical LLMs involves sourcing high-quality, domain-specific data. Collecting this data can be both labor-intensive and costly.
Bias and Fairness: Like their general LLM counterparts, Vertical LLMs can inherit biases present in their training data. Ensuring fairness in outcomes is vital to avoid reinforcing existing societal biases.
Ongoing Maintenance: To remain effective, Vertical LLMs need continual updates in line with evolving terminology and knowledge within specific fields. Keeping these models relevant can be a considerable challenge.
As an AI engineer, it’s crucial to navigate these aspects wisely to develop effective and responsible Vertical LLMs.
Looking to the Future
The future of Vertical LLMs is full of promise. Several trends are likely to shape the development and implementation of these models moving forward:
1. Greater Specialization
We may see Vertical LLMs becoming even more specialized. As industries grow and new markets arise, models that cater to narrower subdomains will likely gain prominence. Over time, we could see models perfectly tailored to tackle niche challenges effectively.
2. Cross-Disciplinary Collaboration
The development of Vertical LLMs will increasingly rely on collaboration between AI engineers and domain experts. Working together ensures that models are not just technically sound but also attuned to the specific needs of each field. This cooperation will be essential for realizing the full potential of these models.
3. Adaptive Learning
Real-time learning capabilities are another emerging trend. Future Vertical LLMs are expected to incorporate adaptive mechanisms that allow them to learn from new data consistently. This feature will enhance their responsiveness and accuracy in dynamic environments.
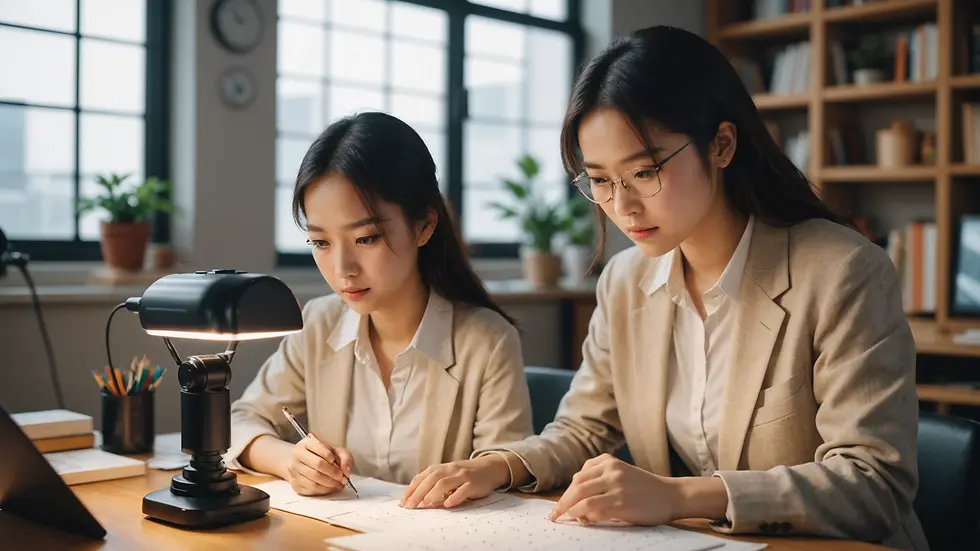
4. Ethical Awareness
As Vertical LLMs develop, addressing ethical considerations will become crucial. AI engineers must prioritize transparency and fairness in these models, ensuring they operate responsibly and do not propagate biases. As trust in AI systems grows, accountability will become increasingly vital.
A Positive Outlook
The rise of Vertical LLMs marks an exciting turning point in AI. These specialized models hold the potential for more accurate, efficient, and context-sensitive applications across various sectors.
As AI engineers, we face challenges such as data limitations and ethical concerns, but these can be managed through collaboration and diligence. The future for Vertical LLMs is bright, and I am eager to see how they will continue to transform the landscape of artificial intelligence in the coming years. I urge fellow AI engineers to explore this exciting field and harness the immense possibilities of Vertical LLMs.
Comments